**Note this is the short summary of the research paper which is available at https://journals.plos.org/ploscompbiol/article?id=10.1371%2Fjournal.pcbi.1006633
Outline
This research paper explains and compares the way of humans perceiving images and trying to replicate through Deep Neural Network (DNN).The basic idea here is to read thoughts of humans through fMRI and then visualize them to create or predict an image.
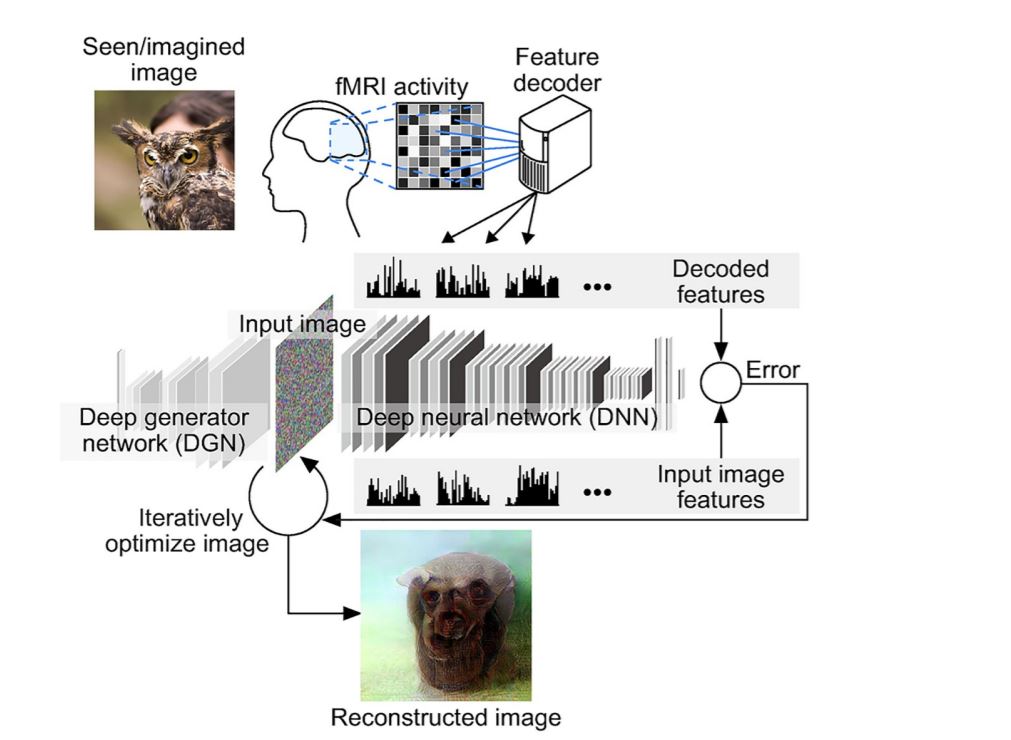
So, it will have a human look at a picture as the example image here shows, that it will measure the MRI activity then it will use a feature decoder in order to map that MRI activity to features of a deep neural network and then they will reconstruct the image that is closest to those features in the neural network and by reconstruction they get out an image of what the human sees.
Process Flow
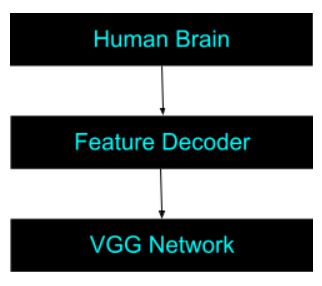
The fMRI machine measure the fMRI activity that means it measures which ones part are active and and measures the blood flow into brain now they need feature decoder but because we want to have these features correspond to features in a neural network the neural network here is vgg there is a bunch of layers and so on. So we have to put the image right into the neural network and then observe its features in the neural network and then we have to put the same image through the human and then you observe the fMRI features.
Results
So after taking all the efforts to reconstruct human visualization the results are shown below
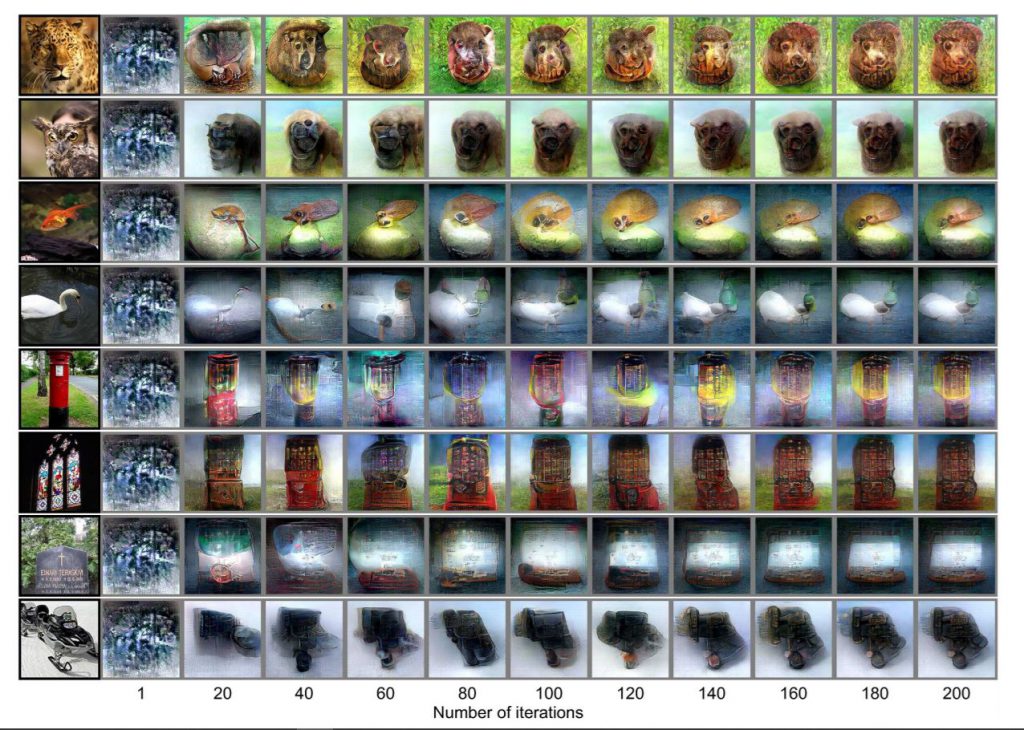
This shows that though they had fMRI data of the human brain the model did not actually produce the result better to recognize at looking once. Though humans were able to predict the original image from the model prediction with accuracy of 95+% but the task for the model did not actually fit well.